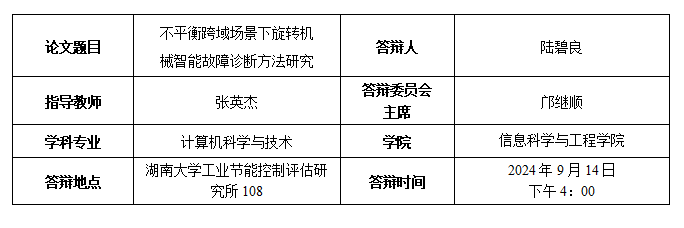
学位论文简介
旋转机械,例如风力涡轮发电机,容易受到多种因素的影响而发生故障,其故障诊断系列关键科学问题被列为“面向国家重大战略需求的基础研究”。因此,本文从源领域和目标领域数据分布的差异、领域间数据不平衡现象构建四种知识迁移故障诊断场景,研究基于不平衡领域迁移的旋转机械智能故障诊断方法。取得了以下主要创新性研究成果:
针对旋转机械故障数据的不平衡领域迁移学习问题,从领域不平衡故障诊断研究的角度出发,对迁移学习所讨论的问题进行明确的定义,从理论角度讨论迁移学习故障诊断泛化误差边界。从方法需求的角度出发,通过经验性的分析多个传统迁移学习诊断方法的跨域诊断性能发现:当训练模型的训练集与测试集来自领域不平衡领域时这些方法的泛化性能均会降低。
以迁移学习故障诊断理论为基础,针对旋转机械故障数据的类间和类内不平衡问题,提出一个改进的域适应模型,称为“类别不平衡感知深度对抗性自适应网络(CIDAA)”。结合5 兆瓦参考传动链模型获得的轴承损伤数据集发现了故障诊断的类间和类内不平衡规律,结果表明,CIDAA 模型可以减少时变环境条件和类别不平衡数据的影响,从而比标准模型实现更高的故障分类准确性。
以类间和类内不平衡迁移学习故障诊断为基础,针对开放集无监督领域适应故障诊断问题,提出基于对抗域自适应的双分类器互学习网络(DCML);通过在两个公开数据集上进行广泛的实验测试,验证所提方法的性能,提出开放集无监督领域适应场景下故障诊断需求及其诊断算法,为实现旋转机械的智能故障诊断提供参考。
以开放集无监督领域适应故障诊断为基础,针对通用集无监督领域适应故障诊断问题,提出基于离群阈值和域不变特性学习(OTDI)的新颖样本选择方法。通过引入离群值阈值学习,同时保持已知类别与离群类别之间的差异。在面向标签空间异构的通用知识迁移故障诊断实验中,证明该方法在解决领域和标签空间不一致性方面的良好性能。
以无监督领域适应故障诊断为基础,针对开放集多源领域泛化故障诊断问题,提出一个多域对比编码(MDCC)框架,用于学习开放集域泛化表示。通过将多源领域的公共类别设置为“正对”,公共类别和私有类别之间设置为“负对”,以同时保留域唯一知识和跨域泛化知识。以两个多域数据集上的实验验证提出的MDCC 框架在开放集域泛化方面的优势。
主要学术成果
1. B. Lu, A. Dibaj, Z. Gao, A. R. Nejad, and Y. Zhang, “A class-imbalance-aware domain adaptation framework for fault diagnosis of wind turbine drivetrains under different environmental conditions,” Ocean Engineering, pp. 116-902, 2024. (对应本文第三章)
2. B. Lu, Y. Zhang, Q. Sun, M. Li, and P. Li, “A dual-classifier mutual learning unsupervised domain adaptation network for intelligent diagnosis of rotary machinery under open-set conditions,” (Under review). (对应本文第四章)
3. 陆碧良,张英杰,孙庆帅,李蒲德,“基于加权对抗域自适应的旋转机械开放集跨域故障诊断,” (审稿中). (对应本文第四章)
4. B. Lu, Y. Zhang, Z. Liu, H. Wei, and Q. Sun, “A novel sample selection approach based universal unsupervised domain adaptation for fault diagnosis of rotating machinery,” Reliability Engineering & System Safety, vol. 240, pp. 109-618, 2023. (对应本文第五章)
5. B. Lu, Y. Zhang, Q. Sun, M. Li, and P. Li, “A novel multidomain contrastive-coding-based open-set domain generalization framework for machinery fault diagnosis,” IEEE Transactions on Industrial Informatics, 2024. (对应本文第六章)
6. B. Lu, Z.-H. Liu, H.-L. Wei, L. Chen, H. Zhang, and X.-H. Li, “A deep adversarial learning prognostics model for remaining useful life prediction of rolling bearing,” IEEE Transactions on Artificial Intelligence, vol. 2, no. 4, pp. 329–40, 2021.
7. B. Lu, A. Dibaj, Z. Gao, A. R. Nejad, and Y. Zhang, “A land-to-floating cross-domain wind turbine gearbox fault detection in different environmental conditions using deep adversarial neural network,” (Under review)
8. Liu, Z.H., Lu, B.L., Wei, H.L., Chen, L., Li, X.H. and Wang, C.T. A stacked auto-encoder based partial adversarial domain adaptation model for intelligent fault diagnosis of rotating machines. IEEE Transactions on Industrial Informatics,17(10), pp.6798-6809, 2020.
9. Zhu, H., Huang, Z., Lu, B., Cheng, F. and Zhou, C. Imbalance domain adaptation network with adversarial learning for fault diagnosis of rolling bearing. Signal, Image and Video Processing, 16(8), pp.2249-2257, 2022.
10. Zhu, H., Huang, Z., Lu, B. and Zhou, C. Bearing remaining useful life prediction of fatigue degradation process based on dynamic feature construction.International Journal of Fatigue, 164, p.107169, 2022.
11. Zhang, Y., Li, M., Zhang, Y., Hu, Z., Sun, Q. and Lu, B. An enhanced adaptive unscented kalman filter for vehicle state estimation. IEEE Transactions on Instrumentation and Measurement, 71, pp.1-12, 2022.
12. Sun, Q., Zhang, Y., Lu, B. and Liu, H. Flow measurement-based self-adaptive line segment clustering model for leakage detection in water distribution networks. IEEE Transactions on Instrumentation and Measurement, 71, pp.1-13, 2022.