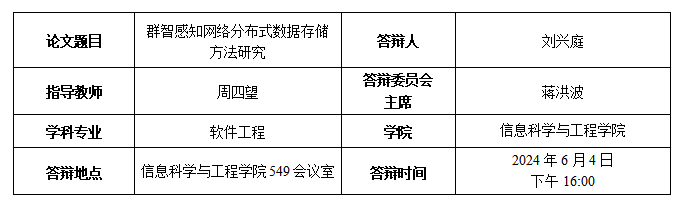
学位论文简介
现有的群智感知系统通常采用基于中央服务器的中心化数据存储管理,充分利用参与者的能力进行数据收集,并确保云服务器能够获取和存储整个目标感知区域的完整信息。然而,由于存储、隐私、网络等各种原因,参与者的传感数据并不一定能正常上传到中央服务器。因此,数据需要分布式存储在网络上,这就产生了一个分布式存储问题。因此,本文旨在研究参与者在目标区域的随机游走,并通过压缩测量值的方式将数据存储在其移动设备上,以实现全局数据的分布式存储在目标网络上。以此为基础,进一步考虑数据质量和成本问题,研究如何在更低成本下得到更高精度的重构数据的分布式存储方案。本研究的主要贡献如下:
(1) 为群智感知系统中的分布式数据存储提出了两个停止准则,以在中央服务器恢复通信后更合理地收集测量,并保证在较低成本下获得高精度的重构数据。
(2) 设计了一种智能分布式存储策略,协助中央服务器巧妙地选择这些测量值,最终在相同的测量资源下获得更高精度的重构数据。
(3) 提出了一种基于群智感知的分块压缩数据存储方案。该方案为恢复局部区域提供了更大的灵活性、招募成本节约和更平滑的重构性能,并且在数据分布不均匀的情况下,采样分配方法进一步提高了重构质量。
(4) 设计了一种自适应采样分配策略和联合去噪机制,显著提高了对全局精度影响最大的信息丰富区块的精度,从而提高了全局重构精度。
主要学术成果
[1] X. Liu, S. Zhou, J. Peng, W. Zhang, D. Tang and K. Li, Stopping Criteria for Distributed Data Storage in Compressive CrowdSensing Systems, in IEEE Internet of Things Journal, vol. 11, no. 7, pp. 11767-11778, 1 April, 2024, doi: 10.1109/JIOT.2023.3330983.(SCI一区,本人一作)
[2] X. Liu, S. Zhou, J. Peng, J. Yu, Y. He and W. Zhang, Adaptive Sampling Allocation for Distributed Data Storage in Compressive CrowdSensing, in IEEE Internet of Things Journal, vol. 11, no. 7, pp. 12022-12032, 1 April, 2024, doi: 10.1109/JIOT.2023.3331848.(SCI一区,本人一作)
[3] X. Liu, S Zhou,J Luo, et al. Region-based compressive distributed storage in Mobile CrowdSensing[J]. Future Generation Computer Systems, 2024, 158: 200-209.(SCI一区,本人一作)
[4] X. Liu, S. Zhou, W. Zhang, T. Dong and K. Li, Privacy-Preserving Truth Discovery for Collabora-tive-Cloud Encryption in Mobile Crowdsensing, in IEEE Systems Journal, doi: 10.1109/JSYST.2023.3274812.(SCI二区,本人一作)
[5] J. Peng, S. Zhou*, L. Ouyang, X. Liu. Volatility-Based Diversity Awareness for Distributed Data Storage of Mobile Crowd Sensing, Computer Networks, 2024 (SCI二区,本人四作)
[6] Peng J, Zhou S, Liu X. Volatility-Based Measurements Allocation for Distributed Data Storage in Mobile Crowd Sensing. IEEE Systems Journal. 2023 Oct 3.(SCI二区,本人三作)