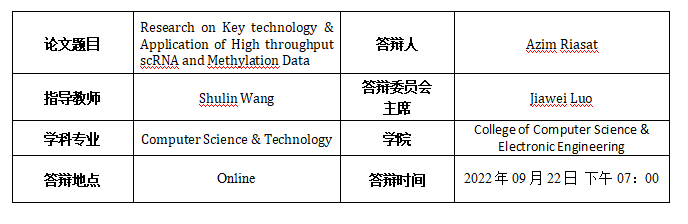
学位论文简介
With the recent advances of the sequencing and high throughput technologies such as microarray and sequence data provide an opportunity to study entire transcriptomes in a smallest detail. To uncover the mystery of important biological question, high throughput technologies play an important role which allows proteomics and transcriptomes data analysis in a rapid pace.
RNA sequencing (RNA-seq) is a genomic approach for the detection and quantitative analysis of messenger RNA molecules in a biological sample and is useful for studying cellular responses. RNA-seq has fueled much discovery and innovation in medicine over recent years. For practical reasons, the technique is usually conducted on samples comprising thousands to millions of cells. RNA sequence has a critical limitation on the analysis of higher noise ratio and heterogeneity analysis because of Bulk RNA sequence is the average of gene expression. While single cell RNA sequence provides a way to gene expression and heterogeneity analysis at the single-cell level.
DNA methylation alteration plays an important role in initiation of different types of cancer and a range of other human diseases. In addition, silencing of downstream tumor suppressor genes is initiated by local hypermethylation and instability of chromosomes is often caused by global hypo-methylation. The improvement of high throughput technologies such as microarray based Infinium HumanMethylation 27 k bradchip, Human Methylation 450K bradchip and MethylationEpic Beadchip microarray 850K enable the identification of cancer biomarkers and therapeutic targets. The assessment of DNA methylation profile helps to understands the function and characteristics of epigenetics in gene expression regulation. Those high throughput technologies produce a large amount of data. The solid tissues collected from clinical setting are highly heterogeneous, those tissue contains various types of cells, such as adjacent normal tissues, cancer cells and stromal cells. The mixed signals form normal and cancer cells often generate complications on biological data analysis. The identification of cancer cell in a solid tumor referring tumor purity is an emerging research area of epigenetics and therapeutic targets recently.
The main research contents and innovations are as follows
1. Due to technical inadequacy, the presence of dropout events hinders the downstream and differential expression analysis. Therefore, it demands an efficient and accurate approach to recover true gene expression. To fill the gap, we present a novel Single-cell RNA drop-out imputation method to retrieve the original gene expression of the genes with excessive zero and near-zero counts. By taking consideration of correlation and negative distance between cells we develop CDSImpute (Correlation Distance Similarity Imputation) to identify drop-outs induced in ScRNA-seq data rather than biological zeros and recover true gene expression. The improvement is consistent with simulation data and several publicly available scRNA-seq datasets.
2. Considering the advances of high-throughput technologies more and more scRNA-seq data sets are available publicly so there is an increasing demand to compare datasets based on their origins. Also, annotation of new samples and identification of similar cells by comparing with reference samples is very crucial for scRNA-seq analysis. The dropout detection algorithm CDSImpute is completely reliant on the similarity detection algorithm so that this dropout detection algorithm is capable of detecting similar cells.
3. Cancer epigenetics is induced not only from individual molecules but also from the dysfunction of the system and the coupling effect of genes. While rapid advances are being made in the development of tools for single-cell RNA-seq data analysis, few slants are noticed in the potential advantages of single-cell network construction. Here, we used network perturbation theory with significant analysis to develop a cell-specific network that provides an insight into gene-gene association based on molecular expressions in a single-cell resolution. Besides, using this method, we can characterize each cell by inspecting how genes are connected and can identify the hub genes using network degree theory. Pathway & Gene enrichment analysis of the identified cell-specific high network degree genes supported the effectiveness of this method. This method could be beneficial for personalized drug design and even therapeutics.
4. Solid tissues collected from patient-driven clinical settings are composed of both normal and cancer cells, which often precede complications in data analysis and epigenetic findings. The Purity estimation of samples is crucial for reliable genomic aberration identification and uniform inter-sample and inter-patient comparisons. Here a simple but effective and flexible method is designed to estimate the level of methylation, which infers tumor purity without prior knowledge from other datasets. Comprehensive analysis of our approach on Illumina Infinium 450 k methylation microarray TCGA Breast Cancer data exhibits improved performance for purity assessment that is highly correlated with other advanced methods.
5. Purity estimation consist of several steps including combined mean- variance score calculation using case control design principal and ranking of hypermethylation and hypomethylation CpG sites. The case control statistical mean variance score can be also effective for cancer and normal samples RNA-sequence analysis and differential expression analysis. Each hypermethylated and hypomethylated CpG sites bound to some specific genes and it can help to have a closer look into suppressor, promoter region and gene body. Gene enrichment analysis and KEGG pathway analysis also conducted on those identified genes.
主要学术成果
[1] R. Azim, S. Wang, “CDSImpute: An Ensemble Similarity Imputation method for single-cell RNA sequence dropouts,” Computers in Biology and Medicine, vol. 146, 105658, Aug. 2022, doi: 10.1016/j.compbiomed.2022.105658. (SCIE, Q1, IF: 6.698)
[2] R. Azim, S. Wang, S. Zhou, and X. Zhong, “Purity estimation from differentially methylated sites using Illumina Infinium methylation microarray data,” Cell Cycle, vol. 19, no. 16, pp. 2028–2039, Aug. 2020, doi: 10.1080/15384101.2020.1789315. (SCIE, Q2, IF: 5.173)
[3] S. Zhou, S. Wang, Q. Wu, R. Azim, and W. Li, “Predicting potential miRNA-disease associations by combining gradient boosting decision tree with logistic regression,” Comput. Biol. Chem., vol. 85, p. 107200, Apr. 2020, doi: 10.1016/j.compbiolchem.2020.107200. (SCIE, Q2, IF: 3.737)
[4] R. Azim and S. Wang, “Cell-specific gene association network construction from single-cell RNA sequence,” Cell Cycle, pp. 1–16, Sep. 2021, doi: 10.1080/15384101.2021.1978265. (SCIE, Q2, IF: 5.173)
[5] Y. Qin, A. B. M. Munibur Rahman, and R. Azim, “Research on innovation and strategic risk management in manufacturing firms,” in Proceedings of the International Conference on Electronic Business (ICEB), 2018, vol. 2018-Decem, pp. 505–521.